LeafPulse
About LeafPulse
During a visit to my grandparents' village, I witnessed a simple yet serious issue affecting farmers: they weren’t sure how much water their crops needed. Walking along the irrigation channels, I saw some fields waterlogged, while others, just a short distance away, lay dry and cracked. The scene highlighted a harsh reality—farmers were relying on guesswork rather than informed decisions to irrigate their fields. As I researched further, I realized that millions of farmers across India face this same daily struggle.
Agriculture consumes nearly 78% of India’s freshwater (Indian Central Water Commission) , yet up to 70% of this water is wasted due to inefficient irrigation practices (Indian Council of Agricultural Research) . This means that nearly 56% of the country’s freshwater reserves are lost. With the majority of India’s population dependent on agriculture, these inefficiencies don’t just threaten individual farmers—they undermine economic stability, food security, and the well-being of millions. To address this issue, I founded LeafPulse.
LeafPulse is an advanced computer-vision solution that empowers farmers to make data-driven irrigation decisions by analyzing plant hydration through the microscopic imaging of stomata. Using the YOLOv8 architecture, I trained the model with over 100 primary-collected micrographs of leaves, classified into open or closed stomata, achieving an accuracy of 92%. I curated the dataset meticulously to avoid bias, capturing balanced samples from the leaf's lower epidermal section, where stomata are denser and more visible. I plucked leaves at different times of the day and extracted thin epidermal peels using precise cuts. To enhance cell visibility, I further stained the peels with Safranin and mounted them with glycerin, ensuring stability for microscopic inspection at 40x magnification.
Training involved binary classification over 600 epochs, using libraries like OpenCV, PyTorch, and Ultralytics’ YOLO module. Cross-validation helped ensure robustness in classification accuracy. LeafPulse also includes a chatbot interface, allowing farmers to access real-time, tailored recommendations. Currently being tested in partnership with agricultural research centers, LeafPulse aims to enhance crop health and promote sustainable water use by turning microscopic data into actionable insights.
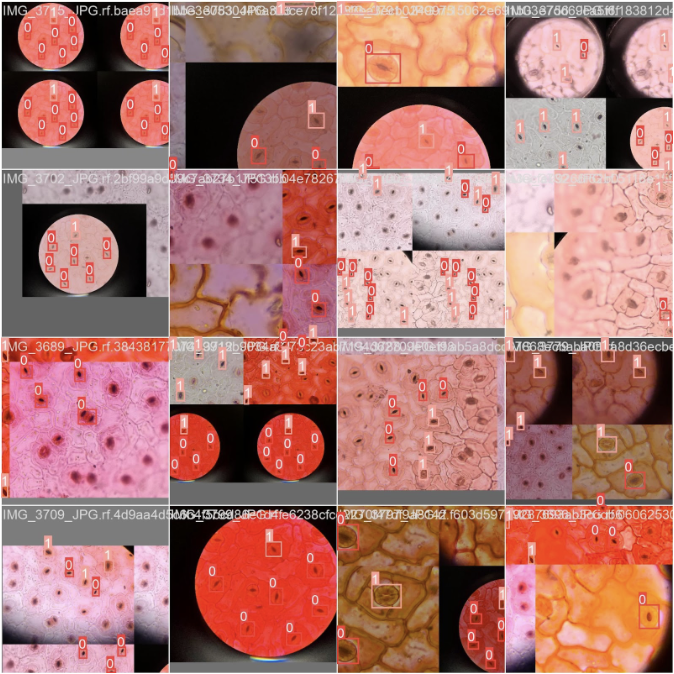
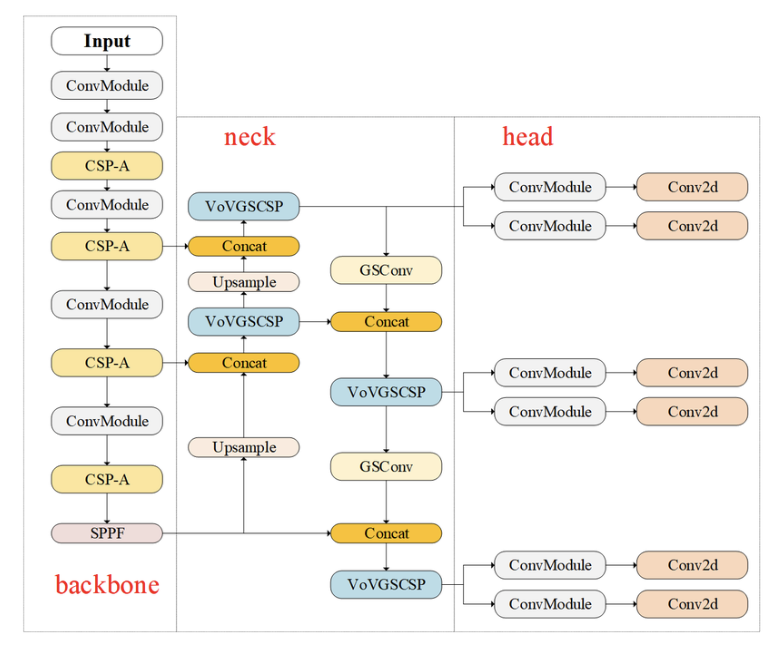
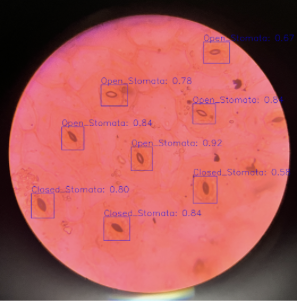
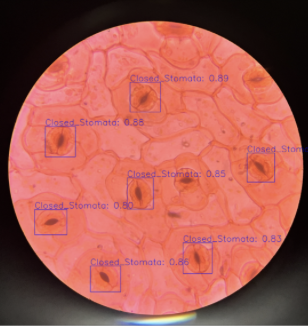
Testing
LeafPulse is undergoing a six-month testing phase at the Rajasthan Agricultural Research Institute (RARI), concluding in November 2024. After this phase, we plan to expand our model to other regions, making it easier for farmers to assess plant water needs and optimize irrigation decisions.
Milestones
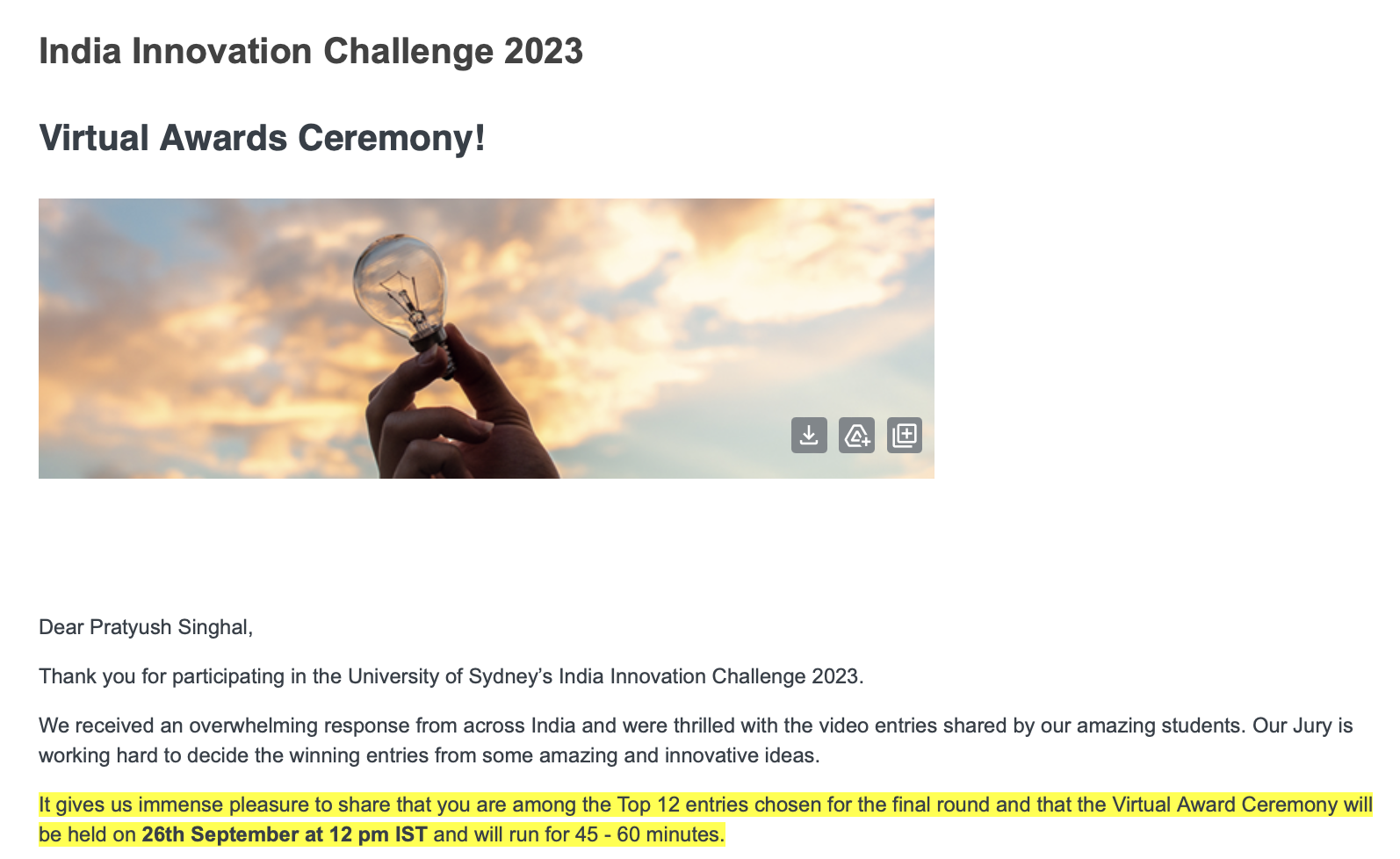
Among Top 12 at India Innovation Challenge 2023
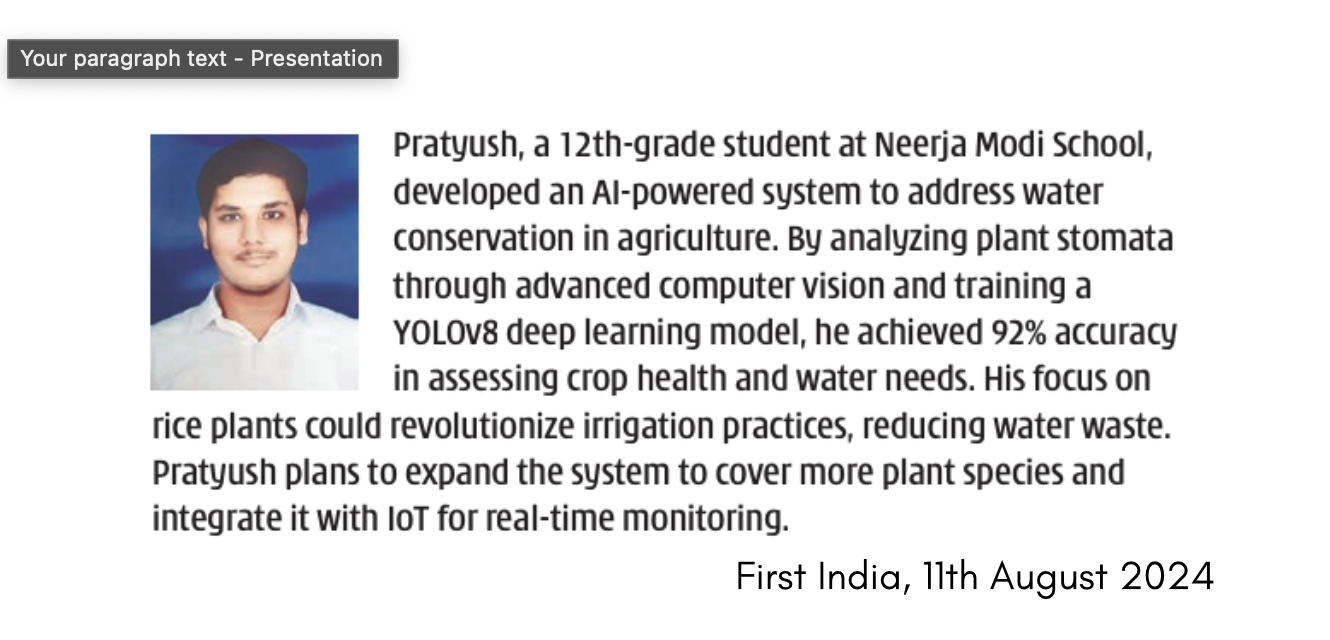
Received recognition from national media channels
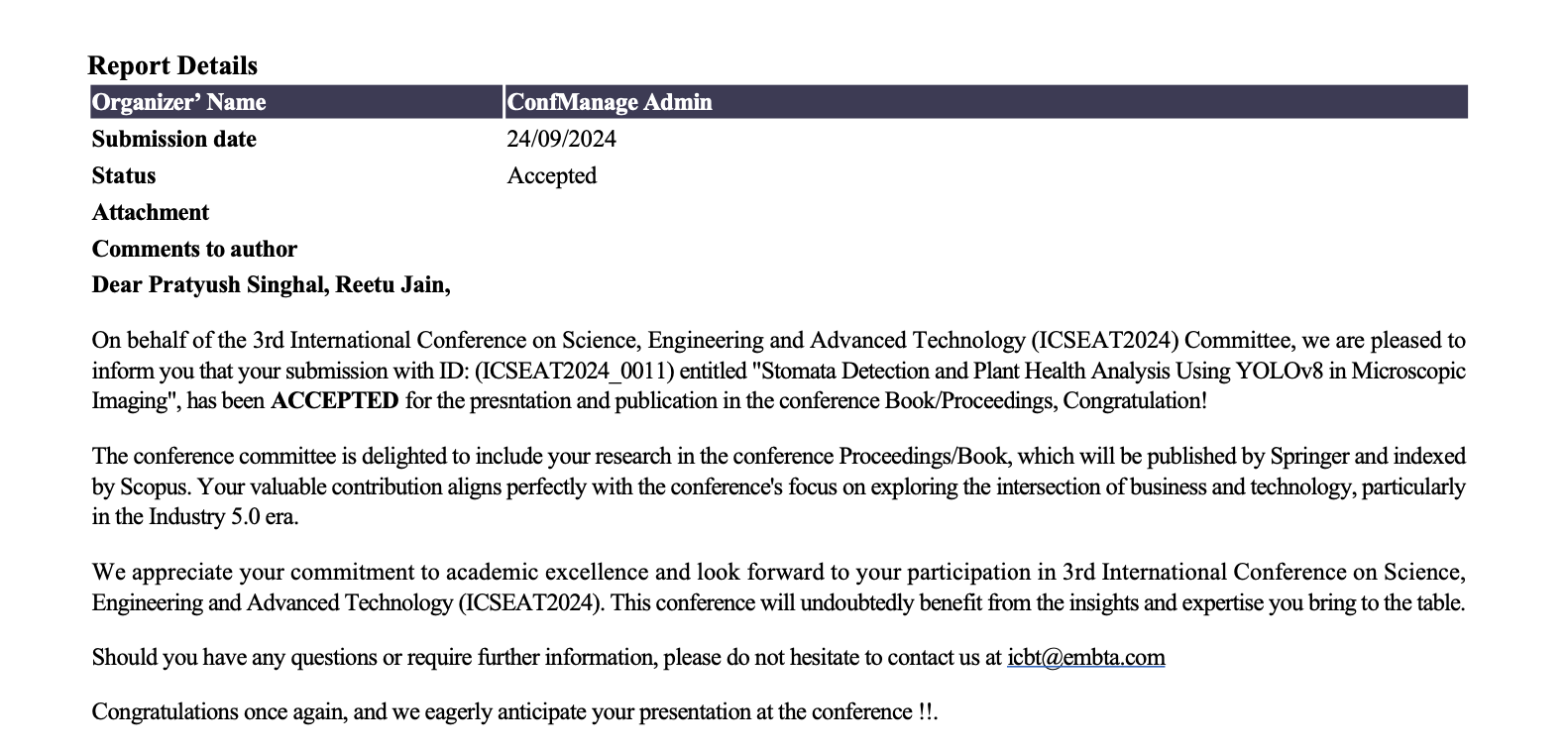
Presented my research to international researchers at ICSEAT 2024. Publishing in SpringerLink